Volume Forecasting for vegetables
A reliable and accurate forecasting tool for predicting the demand for vegetables in the market
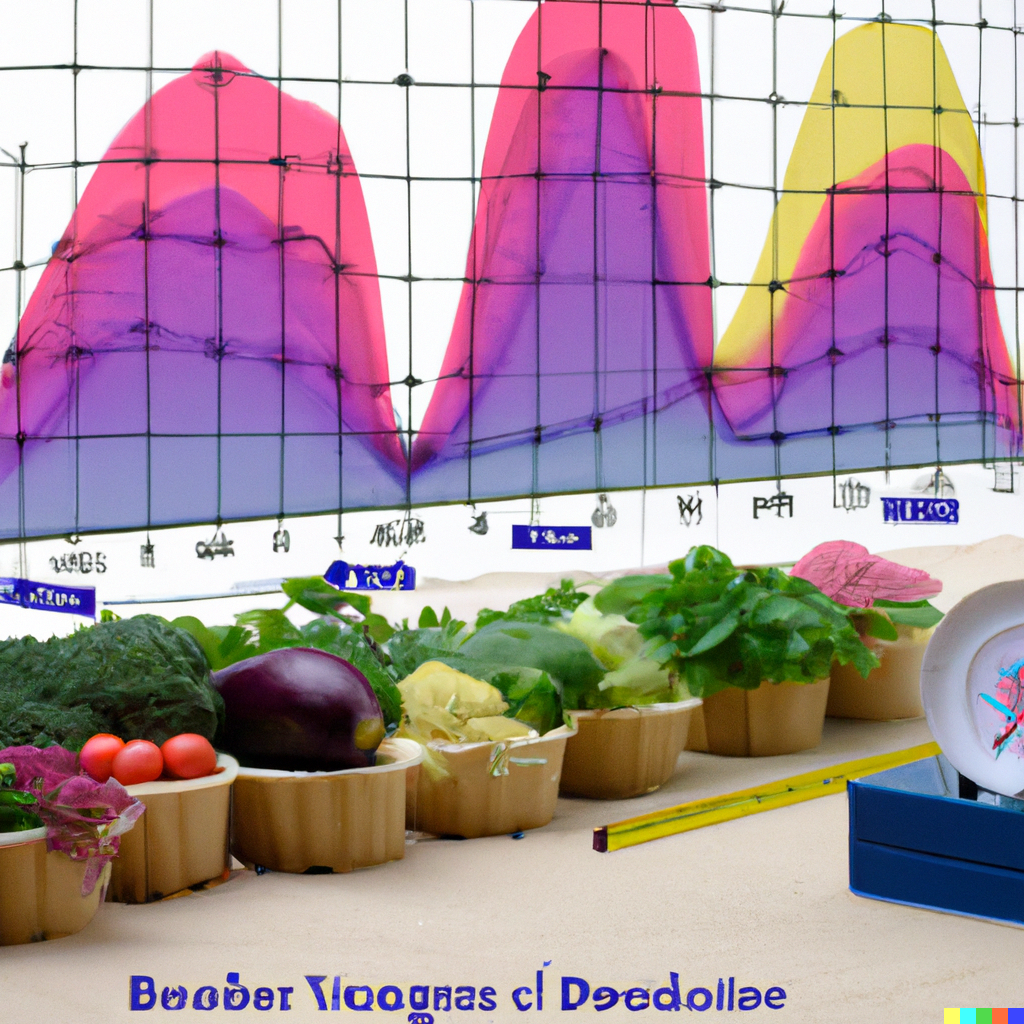
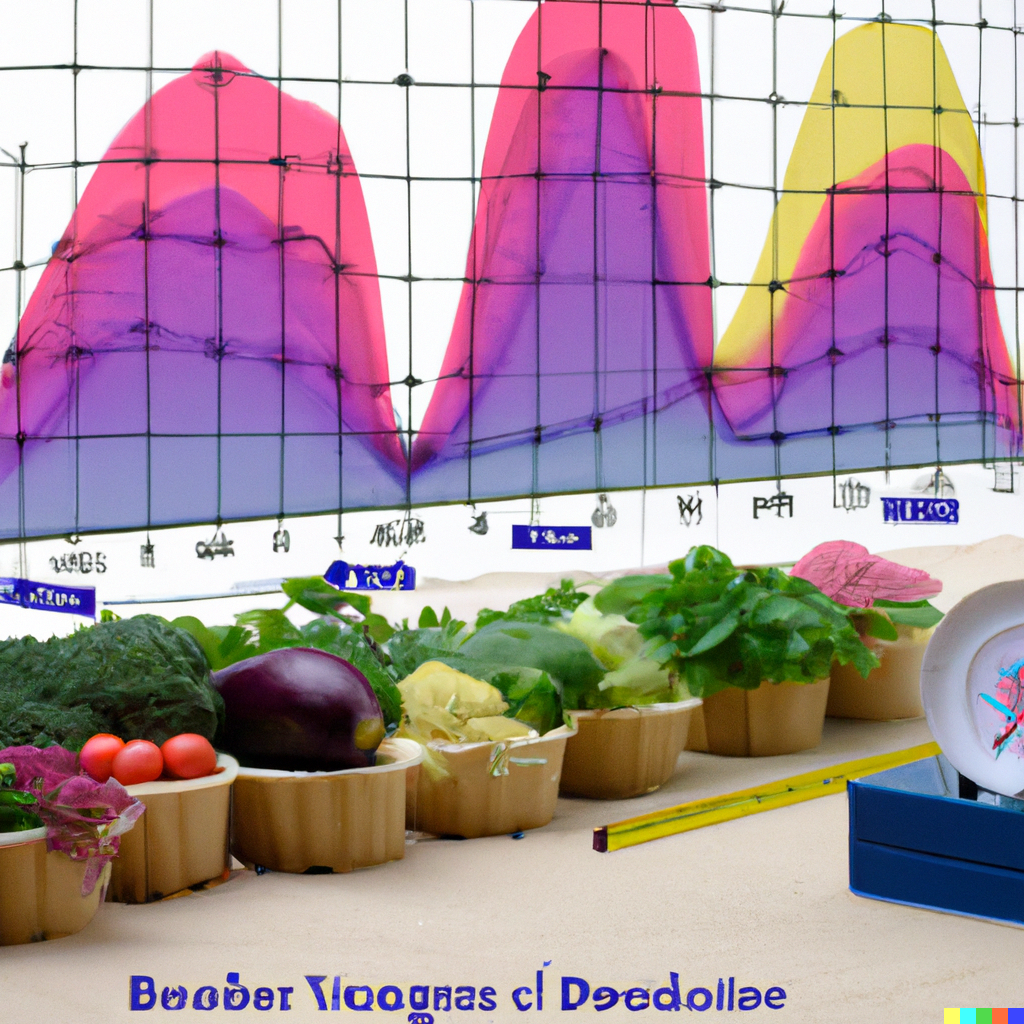
Our aim is to provide a reliable and accurate forecasting tool for predicting the demand for vegetables in the market. By using machine learning techniques, our framework is able to analyze historical data and make informed predictions about future demand.
To implement the framework, we made the following assumptions:
- Accurate and sufficient data on past demand for vegetables is available.
- Demand for vegetables is influenced by a variety of factors, including seasonality, weather, and market trends, which can be captured by relevant features in the data.
- Machine learning models can effectively capture the patterns and trends in the data and make accurate predictions about future demand.
To develop the framework, we followed the following steps:
- Data preprocessing: We cleaned and transformed the data to prepare it for analysis. This involved handling missing or incomplete data, standardizing values, and encoding categorical variables.
- Feature engineering: We selected and created relevant features from the data that could capture the patterns and trends in the demand for vegetables.
- Model selection: We evaluated the performance of different machine learning models using cross-validation and selected the model with the highest accuracy.
- Model training: We trained the selected model on the preprocessed and engineered data to learn the patterns and trends in the data.
- Forecasting: We used the trained model to make predictions about future demand for vegetables based on the input data.
We are confident that our Volume Forecasting Framework for Vegetables will provide a reliable and accurate tool for predicting the demand for vegetables in the market. With the use of advanced machine learning techniques, our framework is able to effectively capture the patterns and trends in the data and make informed predictions about the future.